Exploring DNA Methylation Biomarkers and Deep Learning for Cancer Epigenetics
DOI:
https://doi.org/10.5281/zenodo.11499083Keywords:
DNA Methylation, Cancer Epigenetics, Deep Learning, Biomarkers, Machine Learning, Cancer DiagnosisAbstract
Changes in DNA methylation, such as overall reduced methylation and increased methylation in specific CpG islands, are commonly seen in different types of cancer and also used as biomarkers to detect and diagnose cancer at an early stage. The distinct DNA methylation patterns provide valuable information about cancer progression and therapy. Recent advances in high-throughput techniques such as genome-wide profiling have transformed epigenetics by allowing computational analysis of intricate DNA methylation data. Deep learning techniques have become effective instruments for examining these patterns of methylation, enabling the identification of cancer markers, categorization of tumors, filling in missing data, and forecasting patient survival. This comprehensive review investigates the various uses of deep learning in examining DNA methylation and multi-omics data for cancer studies. It presents state-of-the-art deep learning architectures that are capable of addressing obstacles linked to research on cancer epigenetics. Nevertheless, the review also recognizes possible restrictions and areas for future investigation in this swiftly developing field. This work seeks to improve cancer diagnostics and therapeutic strategies by tackling these challenges and furthering knowledge of epigenetic mechanisms in cancer.
Downloads
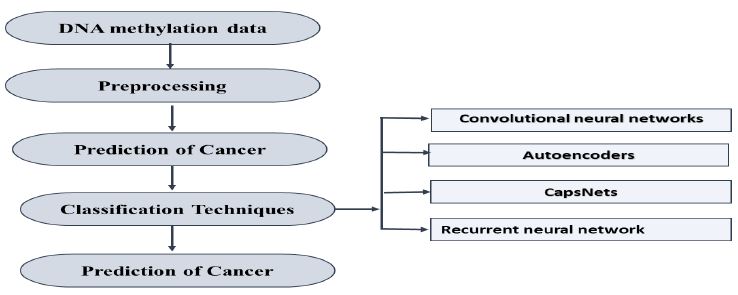
Downloads
Published
How to Cite
Issue
Section
License
Copyright (c) 2024 Remyamol K M, Philip Samuel

This work is licensed under a Creative Commons Attribution 4.0 International License.