Editable Neural Radiance Fields Convert 2D to 3D Furniture Texture
DOI:
https://doi.org/10.5281/zenodo.12662936Keywords:
Neural Radiance, 2D, 3D, TextureAbstract
Our work presents a neural network designed to convert textual descriptions into 3D models. By leveraging the encoder-decoder architecture, we effectively combine text information with attributes such as shape, color, and position. This combined information is then input into a generator to predict new furniture objects, which are enriched with detailed information like color and shape.[1] The predicted furniture objects are subsequently processed by an encoder to extract feature information, which is then utilized in the loss function to propagate errors and update model weights. After training the network, we can generate new 3D objects solely based on textual input, showcasing the potential of our approach in generating customizable 3D models from descriptive text.[2]
Downloads
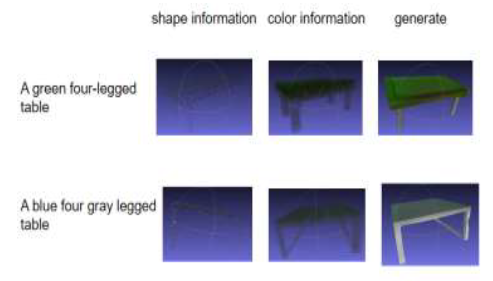
Downloads
Published
How to Cite
Issue
Section
License
Copyright (c) 2024 Chaoyi Tan, Chenghao Wang, Zheng Lin, Shuyao He, Chao Li

This work is licensed under a Creative Commons Attribution 4.0 International License.